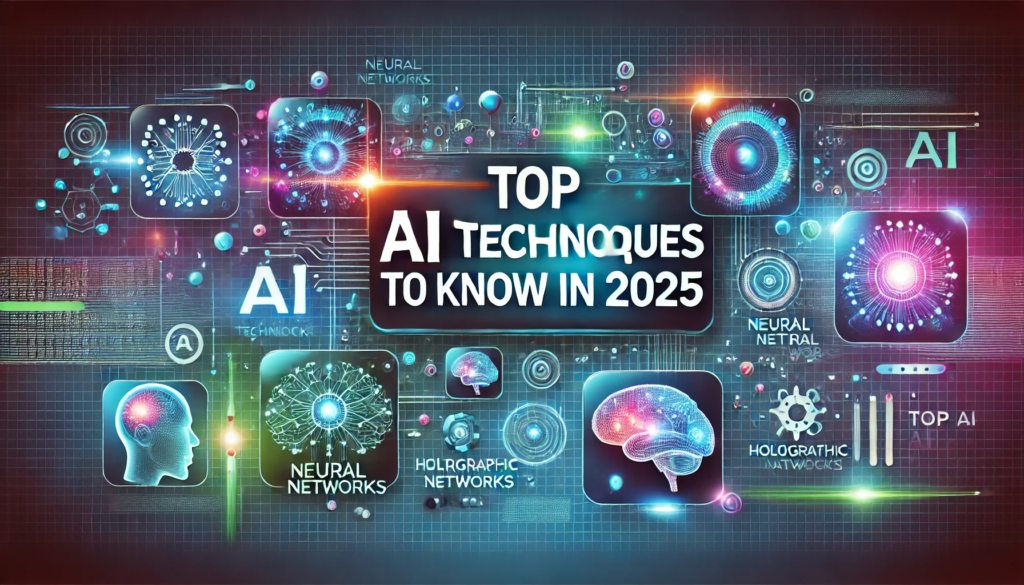
AI Techniques in 2025
Artificial Intelligence (AI) has made tremendous strides over the last few decades, changing the way we live and work. As a result, AI is now redefining many industries and pushing the boundaries of innovation. The techniques that power AI have grown increasingly sophisticated, allowing machines to handle complex tasks that were once considered the exclusive domain of humans.
In this post, I will discuss some of the key AI techniques that are reshaping the field and offering new possibilities.
The Rise of Machine Learning and Deep Learning
Machine learning (ML) is perhaps the most important technique behind the rapid advancement of AI. This approach allows AI systems to learn from data without being explicitly programmed for specific tasks. It is particularly effective in areas like image recognition, natural language processing (NLP), and decision-making. Machine learning algorithms can recognize patterns in large datasets and make predictions based on that data.
Deep learning, a subset of machine learning, takes this concept a step further. Deep learning algorithms use multi-layered neural networks to mimic the way humans process information. These neural networks allow AI to learn more abstract features of data, such as recognizing faces or translating languages. As a result, deep learning has played a crucial role in advancing speech recognition, autonomous vehicles, and even creative fields like music and art.
Although machine learning and deep learning have brought significant improvements in AI, they still require vast amounts of data to function properly. In particular, training deep learning models can be computationally expensive and time-consuming. Nevertheless, these techniques continue to evolve, with researchers focusing on ways to make them more efficient and scalable.
Natural Language Processing (NLP)
Another key area of AI development is Natural Language Processing (NLP). NLP enables machines to understand, interpret, and generate human language. This is an essential component of conversational AI systems, such as chatbots and virtual assistants. NLP techniques allow machines to process text and speech data, identifying meaning, sentiment, and context.
For example, NLP is used in customer service chatbots to understand a customer’s inquiry and respond in a relevant way. NLP can also be found in language translation tools, sentiment analysis applications, and text summarization systems. However, NLP is far from perfect. Machines often struggle with nuances in language, such as sarcasm or ambiguity. As a result, NLP developers are working on improving contextual understanding to make AI interactions more natural.
In the same way that speech recognition has improved over time, NLP systems are becoming more proficient in understanding human language. With the advent of large language models like GPT-3, NLP has entered a new phase of sophistication, enabling machines to generate human-like responses. This has opened up new possibilities for customer support, content creation, and even virtual companionship.
Reinforcement Learning
Reinforcement learning (RL) is another key AI technique that has shown tremendous potential. In this approach, machines learn through trial and error, receiving feedback in the form of rewards or penalties based on their actions. RL is particularly effective for tasks that involve decision-making in dynamic environments, such as game playing, robotics, and autonomous driving.
In comparison to supervised learning, where machines are trained on labeled data, reinforcement learning allows AI systems to learn in real-time by interacting with their environment. For example, RL is used to train self-driving cars to navigate traffic, or to teach robots to perform complex tasks like picking up objects or assembling products.
One of the major challenges of RL is the need for large amounts of data and the risk of trial and error leading to undesirable outcomes. However, as RL algorithms improve, so does their ability to perform complex tasks with greater efficiency and safety.
Generative Models: Creating New Content
Generative models are another fascinating aspect of AI that is helping redefine what machines can do. These models are designed to create new data based on existing data, allowing AI to generate new content such as images, music, or even text. Generative adversarial networks (GANs) are a type of generative model that pits two neural networks against each other: one generates new data, while the other evaluates its authenticity.
Generative models have found applications in various fields. In art, for example, AI-generated art has become a popular trend, with machines creating paintings, digital art, and even sculptures. Similarly, AI has been used in music composition, generating original pieces in various genres. Admittedly, generative models are still evolving, but their potential is enormous.
One controversial area where generative models are making an impact is in the creation of explicit content. NSFW AI chat bots, for instance, can simulate conversations and create content that may not be suitable for all audiences. While such applications raise important ethical and legal concerns, they also highlight the power of generative models in shaping the future of AI and its potential uses.
Generative models also play a significant role in other industries. For instance, in healthcare, AI can generate synthetic medical data for research purposes, reducing the risk of privacy violations. In gaming, AI-generated characters and environments are becoming more realistic, adding to the immersive experience.
AI in the Cloud: The All-in-One Platform
As AI becomes increasingly sophisticated, the demand for powerful computational resources is also growing. Cloud-based platforms offer businesses and developers a way to access AI tools without needing to invest in expensive hardware. Cloud providers like Google, Microsoft, and Amazon offer a wide range of AI services, such as machine learning platforms, natural language processing APIs, and computer vision tools.
The convenience of cloud-based AI is making it easier for companies to integrate AI into their existing infrastructure. Similarly, developers can access a variety of AI tools in one website, allowing them to select the best tools for their specific needs. This all AI tools in one website approach has simplified the process of building and deploying AI solutions, reducing the barriers to entry for businesses of all sizes.
By offering on-demand access to powerful AI resources, cloud platforms have democratized AI development, enabling startups and smaller companies to leverage AI without the need for extensive computational resources. As AI continues to evolve, cloud providers are expected to offer even more advanced services, including automated machine learning and AI-powered analytics tools.
Ethical Considerations and AI Regulation
As AI techniques become more powerful, ethical considerations are becoming increasingly important. AI systems have the potential to revolutionize industries, but they also pose risks, such as job displacement, privacy violations, and unintended consequences. Consequently, policymakers, researchers, and AI developers must work together to create regulations that ensure AI is used responsibly.
Ethical concerns also arise when it comes to AI-generated content. While generative models can create impressive artwork and music, they can also be used to create harmful or misleading content. For example, AI can generate fake news articles or deepfake videos, which can have serious consequences for individuals and society as a whole. It is crucial that AI developers build safeguards into these systems to prevent misuse.
Moreover, as AI systems become more integrated into our lives, the question of accountability arises. Who is responsible when an AI system makes a mistake or causes harm? To address these concerns, it is essential to establish clear guidelines and regulations for AI development, ensuring that these technologies are used ethically and transparently.
The Future of AI Techniques
Looking ahead, the future of AI techniques is bright, with numerous advancements on the horizon. One area that shows great promise is the development of explainable AI (XAI). Unlike traditional AI models, which often operate as “black boxes,” XAI aims to create models that are transparent and can explain their decision-making process. This will be particularly important in fields like healthcare and finance, where understanding the reasoning behind an AI’s decision is crucial.
Furthermore, AI systems will continue to become more specialized, with models being trained to handle specific tasks with greater precision. As AI becomes more integrated into daily life, we can expect to see even more innovative applications, from autonomous transportation to AI-powered healthcare solutions.
Conclusion
The techniques behind artificial intelligence are rapidly evolving, and their potential applications are vast. From machine learning and deep learning to natural language processing and generative models, AI is redefining what machines can do.
However, as AI becomes more powerful, it is essential to address the ethical and regulatory challenges that arise. With the right safeguards in place, AI has the potential to transform industries, improve lives, and shape the future in ways we can only begin to imagine.